Rainfall in general is a complex phenomenon to describe and forecast. Rainfall in tropics is admittedly more complex due to high spatio-temporal variability which is especially true in case of convective precipitation events. This makes it challenging to accurately quantify the amount of rainfall on the ground at any given location without using a rain gauge after an event and even more challenging to forecast rainfall with a significant lead time and accuracy.
Rainfall radars, such as S-, C- and X-band radars, can be used to provide spatially distributed rainfall data anywhere on a map, however, the data is typically measured between 1 and 3 kilometres from the ground. Rain gauges on the other hand can measure rainfall data at ground level, but only at one location and are sparsely distributed. Thus, if rain gauges are not installed densely, they are typically not able to detect spatial distribution of rainfall at the ground level.
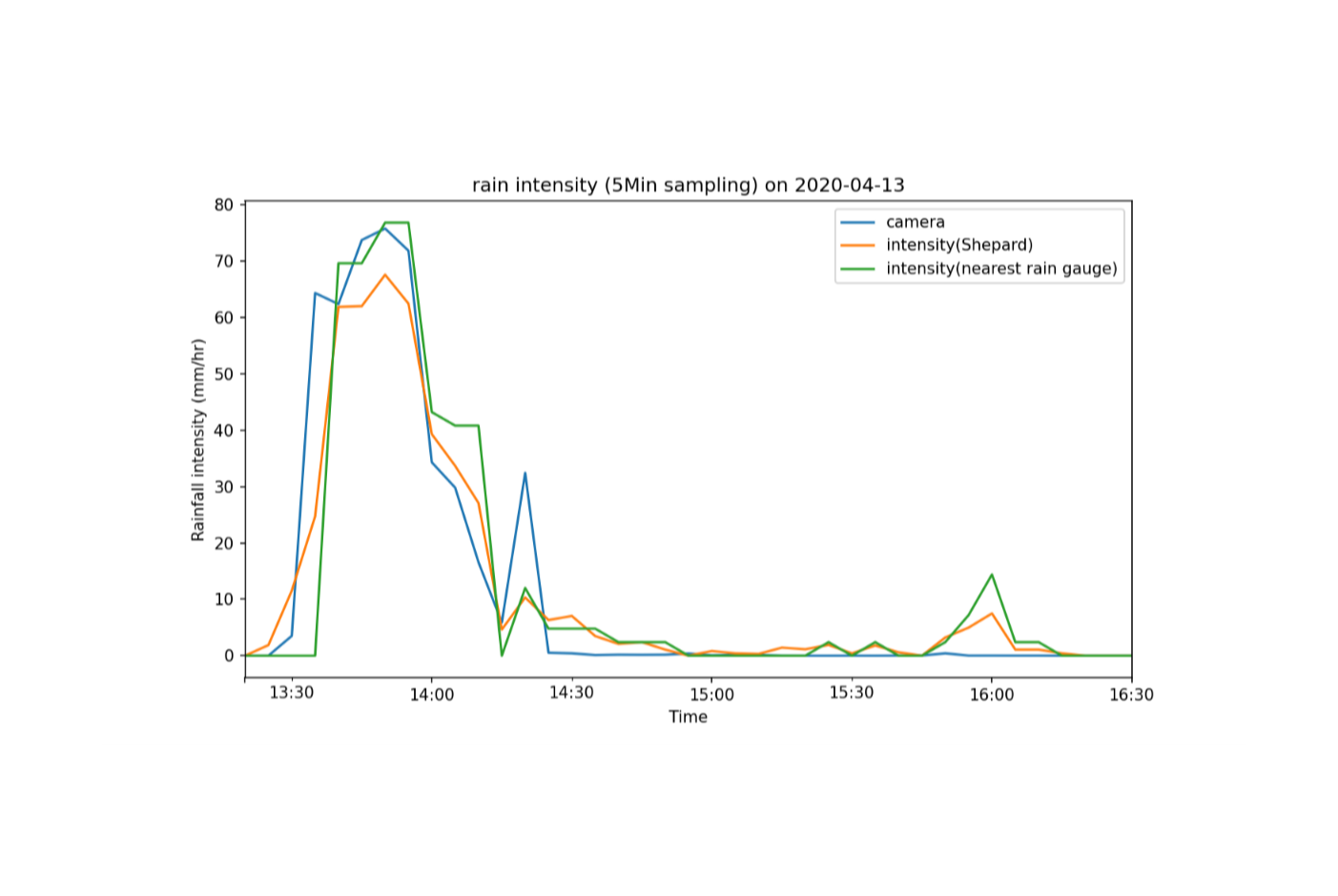
However, CCTVs are everywhere and are densely distributed at ground level; if they can be converted into a rainfall measuring device, valuable highly spatially distributed rainfall data at ground level is within reach.
H2i has collaborated with PUB, Singapore’s National Water Agency to investigate how accurate rainfall can be derived from CCTV footage in real-time. The results are promising; even though the resolution of CCTVs is low (480×320 pixels), a Deep Learning model based on VGG19 (a convolutional neural network model) was able to detect rainfall at places where the rain gauges were not able to detect this rainfall, simply because rain gauges are far more sparsely distributed than CCTVs. For these CCTVs, the results were presented in rainfall categories (no rain, light rain, heavy rain) and compared with a nearby rain gauge.
Tests with a high-resolution (Full HD) IoT device resulted in even more interesting results. The high-resolution footage made it possible to detect rainfall rates in mm/h based on scientifically derived algorithms that count raindrops. However, it should be noted that this is only possible if the rain streaks are clearly visible in footage, which means high quality hardware (i.e. lens) is required. An example is shown in the figure above (where ‘camera’ is the IoT device result, ‘nearest rain gauge’ is the measured rainfall rates at the nearest rain gauge and Shepard a weighted average of four rain gauges around the IoT device).
These results give confidence that CCTVs can be potential rainfall measurement sensors, whose measurements can be combined with other rainfall measurement sensors such as rainfall radars and rain gauges to produce highly accurate spatial and temporal rainfall rates.